The below article is authored by Anurag Sinha, Co-Founder & Managing Director, Wissen Technology
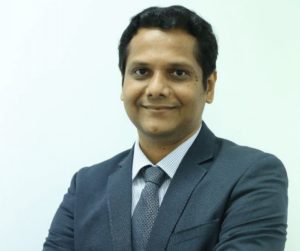
With digital transformation, the adoption of cloud technologies, and the increase in online services being offered across all sectors, data engineering and its management are fast becoming a priority in IT. Since cloud technologies have also allowed for the easy migration of big data from on-premise to online, data is being accessed, processed, generated, and analyzed more than ever before.
Analysis of data and metric dashboards is fast becoming a norm for optimized workflow management, trend forecasts, and data-driven decision-making at all enterprise levels. Here are the top data science predictions for 2023.
Data as a Service
With cloud adoption becoming the default technology option across all sectors, vendors are now offering DaaS (data as a service) as one of the ‘pay as you go’ features. DaaS offers the advantage of access to sophisticated tools and technologies for data management, higher cost savings, better maintenance, and flexibility, along with improved functionality across the enterprise. In essence, tools and technologies are offered to the customer to effectively store, manage, analyze and share big data.
Using DaaS, companies such as those operating in the financial services domain can share their data, analysis models, trends, IPs, and insights with other organizations in the same industry. As it stands, infrastructure management experts are being on-boarded to manage the DaaS model effectively on behalf of the customer.
Artificial Intelligence and Machine Learning
Massive storage capacities in the cloud, the advancement of data management tools, and the migration of big data of enterprises to the cloud have allowed for technologies like AI/ML to be introduced in data engineering. AI and ML are effective technologies that are based on the amount and quality of data that can be processed; the more the data, the better the outcomes.
Big data in the cloud is now being processed in gigantic leaps and bounds – hence unearthing the full capabilities of ML and AI. 2023 is going to see much more data-driven decision-making based on enterprises using sophisticated tools powered by AI and ML. The generation of accurate trend data, forecasting models, automated forensic detection, and mitigation will steadily increase. Partnerships with AI and ML solution providers will be a priority in 2023 to meet the demands of this growing trend.
Edge Computing
Moving data and infrastructure to the edge of the cloud is fast becoming a popular option across vendors and enterprises. Storage of data, processing, and subsequent analysis becomes more effective when the cloud infrastructure is in proximity to the source of the data generation.
With the increase of IoT devices and endpoints spread across vast geographical distances with varying internet infrastructure, the adoption of edge computing along with data collocation at the edge of the cloud will be seen as the logical techno-driven path to follow.
Edge computing will require the IT teams to collaborate with cloud specialists to reap maximum benefit from the edge framework.
Data Fabric and DataOps
Data Fabric is a cloud-based architecture that creates a virtual amalgamated data layer across the cloud. All data, along with its components, is added to this layer with a common entry point to the layer via connectors. This common data layer ensures there are no data silos across the enterprise, thus ensuring better management of data as well as increasing the quality of data that has been amalgamated in this common data layer. Data in this fabric is available easily to any application via connectors regardless of the form, size, structure, or location of the data being accessed.
An emerging operational practice called DataOps is one of the components of the data fabric where data-specific processes, architectures, protocols, technologies, and methodologies are adopted for data management. DataOps is applied to the data pipeline, which connects the application to the data fabric.
Data Clean Rooms
A data clean room is a secure environment where enterprises share their proprietary and/or confidential data with third parties for purposes of joint analysis. This sharing of data is within a strict framework of existing protocols of privacy, data governance, and cyber security.
With data clean rooms, enterprises can collaborate with data safely and securely. A popular example of data clean rooms is to link customer data with that of advertising and branding for trend analysis and forecasting.
Data Analytics Automation
Many data analytics tasks that are manually done by data science engineers can be automated since the tasks are repetitive and can be pre-planned in advance. Data analytics automation bots are being introduced into data processing to complete an entire data analytics life cycle. These automation tools are being enhanced with AI and ML technologies.
With the help of this intelligent automation, big data is being analyzed faster, allowing for better insights, patterns, trends, and data-driven decision-making. Data analytics automation is cutting costs, reducing errors, and increasing the efficiency of the data engineering teams. Vendors offering RPA and intelligent automation expertise are assets in this growing trend of data analytics automation.
Data Science and the Competitive Edge
Trends for 2023 clearly show that companies wanting to gain an edge over their competitors will need to take full advantage of the data at their disposal. Effective cloud adoption, analytics automation, AI / ML, and DataOps are likely indicators of success in 2023.
The adoption of data science techniques and technologies to make realistic data-driven decisions based on insights, forecasts, and patterns of collected data will come as a mandatory brief for any online business.